使用chinese-roberta-wwm-ext预训练模型进行情感分类
餐饮服务评价情感倾向分析
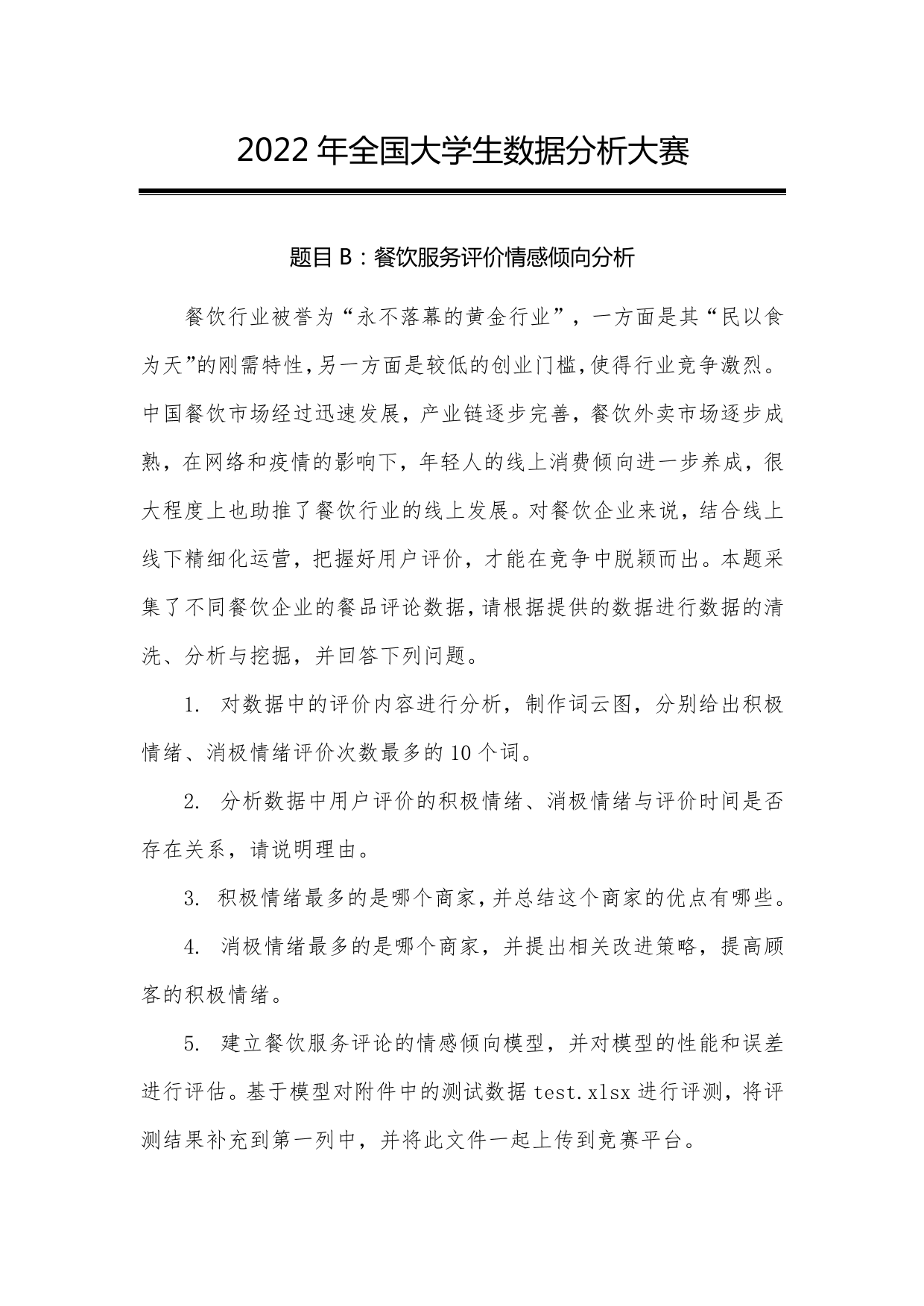
· 建立餐饮评论情感倾向模型
在建立模型前,需对数据进行分析,由于每个商家的评论长度长短不一,所以应该选取一个合适的长度,大于改长度的进行截断,小于该长度的进行填充,评论长度查看如代码所示:
1 2 3 4 5 6 7 8
| token_lens = [] for txt in train.comment: tokens = tokenizer.encode(txt, max_length=512) token_lens.append(len(tokens))
sns.distplot(token_lens) plt.xlim([0, 150]); plt.xlabel('Token count');
|
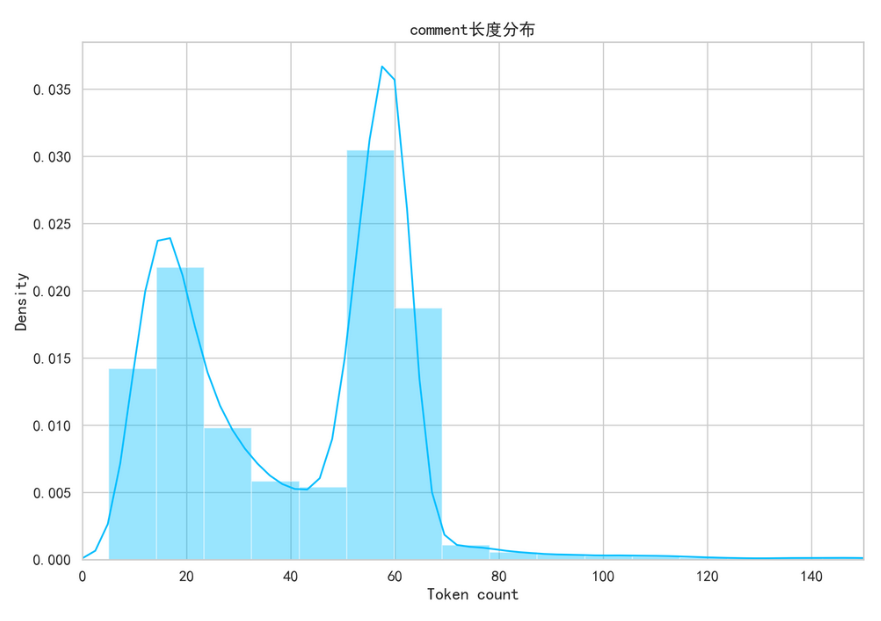
通过上图可以看出文本每条评论的长度绝大多数都在 80 以内,所以可以选取 80 为合适的长度,既不会丢失太多信息,也不会填充太多无用的信息。接下来进行数据集的分割,将训练数据分割成训练集和验证集,训练集用来训练模型,验证集用来评估模型的好坏,将数据的 9 份用来训练,1 份用来验证。如代码所示。
1 2
| df_train, df_test = train_test_split(train, test_size=0.1, random_state=RANDOM_SEED) df_val, df_test = train_test_split(df_test, test_size=0.5, random_state=RANDOM_SEED)
|
由于 comment 内容全为文字内容,无法直接将其输入网络,故应进行数据预处理操作,即文本向量化操作,使用预训练 roberta 对文本进行 embedding,如代码所示:
1 2 3 4 5 6 7 8 9 10 11 12 13 14 15 16 17 18 19 20 21 22 23 24 25 26 27
| class EnterpriseDataset(Dataset): def __init__(self,texts,labels,tokenizer,max_len): self.texts=texts self.labels=labels self.tokenizer=tokenizer self.max_len=max_len def __len__(self): return len(self.texts) def __getitem__(self,item): text=str(self.texts[item]) label=self.labels[item] encoding=self.tokenizer.encode_plus( text, add_special_tokens=True, max_length=self.max_len, return_token_type_ids=True, pad_to_max_length=True, return_attention_mask=True, return_tensors='pt', ) return { 'texts':text, 'input_ids':encoding['input_ids'].flatten(), 'attention_mask':encoding['attention_mask'].flatten(), 'labels':torch.tensor(label,dtype=torch.long) }
|
上述编码的结果包含:input_ids和attention_mask,其中input_ids为编码的结果,attention_mask为可以保证模型在做attention时,有效数据不会被mask。
接下来是模型的搭建,通过搭建神经网络模型,进行数据的预测,本赛题使用的模型是chinese-roberta-wwn模型,该模型在情感分类任务上较为优越,下面是模型的加载,如代码所示。
1 2 3
| PRE_TRAINED_MODEL_NAME = 'hfl/chinese-roberta-wwm-ext' tokenizer = AutoTokenizer.from_pretrained(PRE_TRAINED_MODEL_NAME)
|
在chinese-roberta-wwn模型的后添加全连接层进行二分类处理,即积极情绪和消极情绪的分类,同时,为防止过拟合,选择神经网络的丢弃率为0.3,网络搭建的实现如代码所示。
1 2 3 4 5 6 7 8 9 10 11 12 13 14 15
| class EnterpriseDangerClassifier(nn.Module): def __init__(self, n_classes): super(EnterpriseDangerClassifier, self).__init__() self.bert = BertModel.from_pretrained(PRE_TRAINED_MODEL_NAME) self.drop = nn.Dropout(p=0.3) self.out = nn.Linear(self.bert.config.hidden_size, n_classes) def forward(self, input_ids, attention_mask): _, pooled_output = self.bert( input_ids=input_ids, attention_mask=attention_mask, return_dict = False ) output = self.drop(pooled_output) return self.out(output)
|
对于神经网络模型,需要选择合适的优化器,以及损失函数的选取。这里选择的优化器为AdamW,其优点是在Adam优化器的基础上加入$L_0$正则化,有效避免了过拟合问题。损失函数选取的是交叉熵损失函数,用于评估分类问题。如代码所示:
1 2 3 4 5 6 7 8
| optimizer = AdamW(model.parameters(), lr=2e-3, correct_bias=False) scheduler = get_linear_schedule_with_warmup( optimizer, num_warmup_steps=0, num_training_steps=total_steps ) loss_fn = nn.CrossEntropyLoss().to(device)
|
· 二 情感倾向模型的训练和评估
在神经模型建立完成之后,需要进行模型的训练,如代码所示。
1 2 3 4 5 6 7 8 9 10 11 12 13 14 15 16 17 18 19 20 21 22 23 24 25 26 27 28 29 30 31
| def train_epoch( model, data_loader, loss_fn, optimizer, device, scheduler, n_examples ): model = model.train() losses = [] correct_predictions = 0 for d in data_loader: input_ids = d["input_ids"].to(device) attention_mask = d["attention_mask"].to(device) targets = d["labels"].to(device) outputs = model( input_ids=input_ids, attention_mask=attention_mask ) _, preds = torch.max(outputs, dim=1) loss = loss_fn(outputs, targets) correct_predictions += torch.sum(preds == targets) losses.append(loss.item()) loss.backward() nn.utils.clip_grad_norm_(model.parameters(), max_norm=1.0) optimizer.step() scheduler.step() optimizer.zero_grad() return correct_predictions.double() / n_examples, np.mean(losses)
|
训练完成后需进行模型的评估,选择所给数据的五分之一用来模型的评估,并计算相关的准确率,进行可视化展示,如代码所示。
1 2 3 4 5 6 7
| plt.plot(torch.tensor(history['train_acc'], device='cpu'), label='train accuracy') plt.plot(torch.tensor(history['val_acc'], device='cpu'), label='validation accuracy') plt.title('Training history') plt.ylabel('Accuracy') plt.xlabel('Epoch') plt.legend()
|
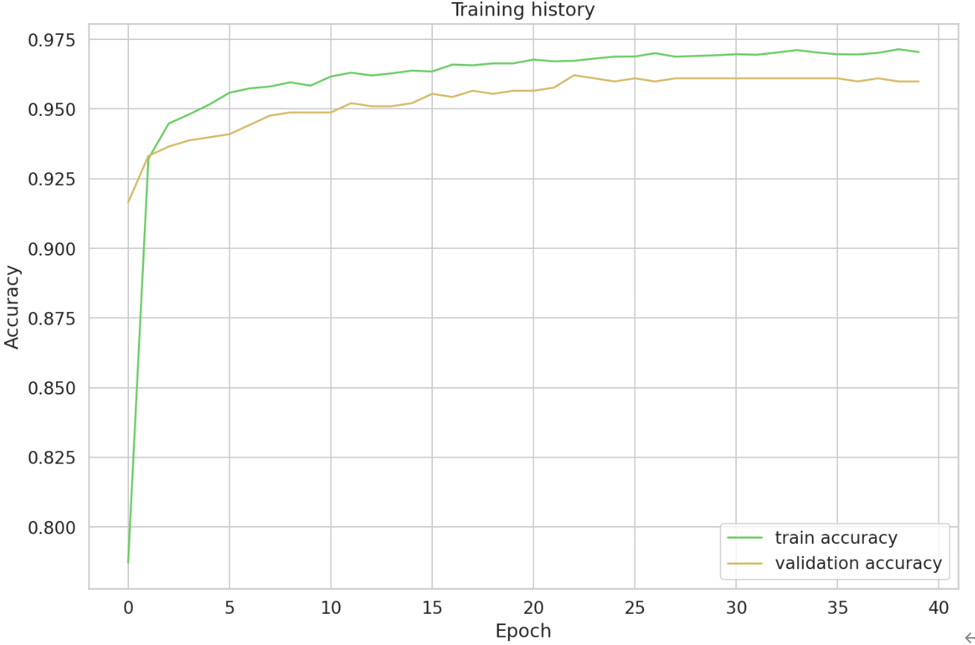
从上图可以看出,训练准确率和验证准确率都在增高,最终训练准确率收敛在97.2%左右
接下来查看一下模型的混淆矩阵,如代码所示。
1 2 3 4 5 6 7 8 9 10
| def show_confusion_matrix(confusion_matrix): hmap = sns.heatmap(confusion_matrix, annot=True, fmt="d", cmap="Blues") hmap.yaxis.set_ticklabels(hmap.yaxis.get_ticklabels(), rotation=0, ha='right') hmap.xaxis.set_ticklabels(hmap.xaxis.get_ticklabels(), rotation=30, ha='right') plt.ylabel('True label') plt.xlabel('Predicted label'); cm = confusion_matrix(y_test, y_pred) df_cm = pd.DataFrame(cm, index=class_names, columns=class_names) show_confusion_matrix(df_cm)
|
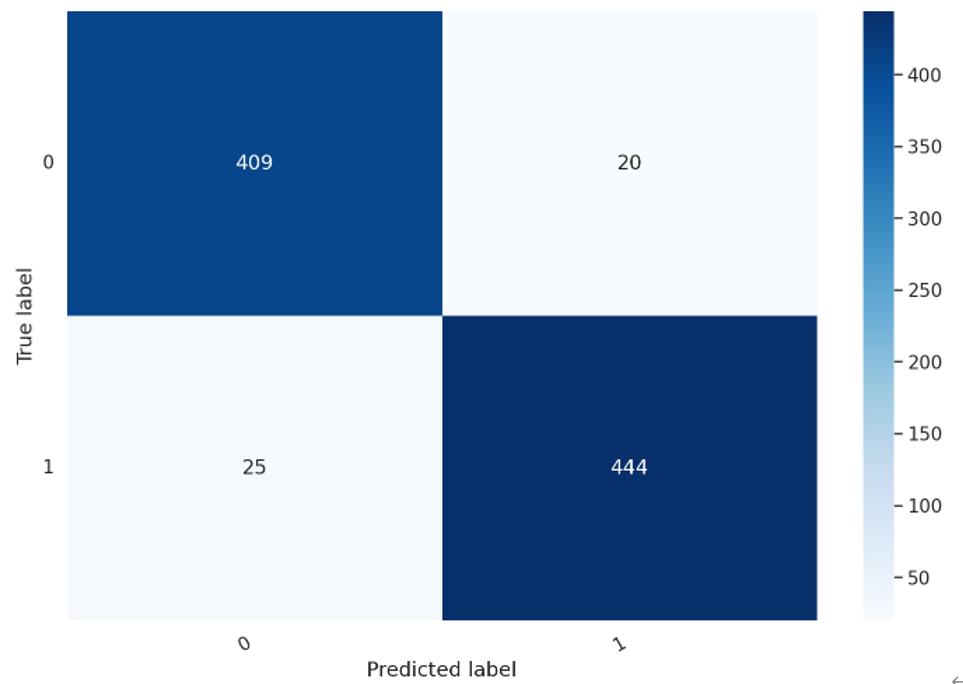
从以上混淆矩阵可以观察出,TF为409,TP为444,在验证数据中,共有853条样本预测正确,45条样本是预测错误的,准确率在97%左右。接下来看一下模型各方面的评估。如代码所示。
1
| print(classification_report(y_test, y_pred, target_names=[str(label) for label in class_names]))
|
|
Precision |
Recall |
F1-score |
Support |
0 |
0.94 |
0.95 |
0.95 |
429 |
1 |
0.96 |
0.95 |
0.95 |
469 |
Weighted avg |
0.95 |
0.95 |
0.95 |
898 |
Maacro avg |
0.95 |
0.95 |
0.95 |
898 |
· 三 对附件test.xlsx进行预测
首先对测试集进行读取,发现测试集共含有1500条样本,由于已经完成了模型的训练和评估步骤,接下来可以进行模型的预测,并将结果补充到文件的第一列。如代码所示。
1 2 3 4 5 6 7 8 9 10 11 12 13 14 15 16 17 18 19
| def con(sample_text): encoded_text = tokenizer.encode_plus( sample_text, max_length=MAX_LEN, add_special_tokens=True, return_token_type_ids=False, pad_to_max_length=True, return_attention_mask=True, return_tensors='pt', ) input_ids = encoded_text['input_ids'].to(device) attention_mask = encoded_text['attention_mask'].to(device) output = model(input_ids, attention_mask) _, prediction = torch.max(output, dim=1) return class_names[prediction] test['target'] = test['comment'].apply(lambda x:con(x))
|